Forecasting incidence of hepatitis A with ARIMA-GRNN hybrid model in China
-
摘要: 目的 探讨ARIMA-GRNN组合模型在甲肝发病序列预测中的应用,并比较其与ARIMA模型和BPNN模型的预测效果。方法 通过收集2004年1月~2014年12月我国甲肝发病序列资料,用SPSS 13.0建立ARIMA模型,用Matlab 8.0建立BPNN模型和ARIMA-GRNN组合模型,并用2014年数据对模型的预测效果进行评价。结果 针对我国甲肝发病序列建立的三种预测模型拟合的平均相对误差的值依次为:ARIMA模型(7.29 785)
结论 ARIMA-GRNN组合模型的拟合及预测效果优于ARIMA模型和BPNN模型。 Abstract: Objective To explore the application of ARIMA-GRNN hybrid model, ARIMA model and BPNN model in the prediction on incidence of hepatitis A in China and compare the predicative effect among them. Methods The data of incidence of hepatitis A from January 2004 to December 2014 in China was collected and SPSS 13.0 was used to construct ARIMA model, Matlab 8.0 was used to establish BPNN model and ARIMA-GRNN hybrid model, afterwards the data in 2014 was used to evaluate the effect of prediction. Results The average relative error of the three models, which was established to predict the incidence of hepatitis A sequence in China, fitness was 7.29785, 5.86174 and 4.91063 for ARIMA model, BP network model and ARIMA-GRNN hybrid model, respectively. The average relative error of accuracy predicted by ARIMA model, BP network model and ARIMA-GRNN hybrid model in 2014 was 6.44 067, 5.74 400 and 4.86 292, respectively. The results of other model evaluation indices, such as average error rate, mean square predict error and mean absolute error, also showed that ARIMA-GRNN hybrid model had the minimum error. Conclusions ARIMA-GRNN combination model is superior to the ARIMA model and BPNN model.-
Key words:
- Models, Statistical /
- Hepatitis A /
- Incidence /
- Forecasting
-
鲁婧婧,谈晔,陈秀云,等. ARMA模型用于预测病毒性甲型肝炎发病趋势 [J]. 中华疾病控制杂志, 2014,18(4):346-350. Guan P, Huang DS, Zhou BS. Forecasting model for the incidence of hepatitis A based on artificial neural network [J]. World J Gastroenterol, 2004,10(24):3579-3582. 张兴裕,周丽君,刘元元,等. SARIMA模型与残差自回归模型在甲肝发病率预测中的应用及比较 [J]. 现代预防医学, 2012,39(5):1065-1067,1074. Zhou L, Yu L, Wang Y, et al. A hybrid model for predicting the prevalence of schistosomiasis in humans of Qianjiang City, China [J]. PLoS One, 2014,9(8):e104875. Yan W, Xu Y, Yang X, et al. A hybrid model for short-term bacillary dysentery prediction in Yichang City, China [J]. Jpn J Infect Dis, 2010,63(4):264-270. Liu L, Luan RS, Yin F, et al. Predicting the incidence of hand, foot and mouth disease in Sichuan province, China using the ARIMA model [J]. Epidemiol Infect, 2016,144(1): 144-151. 王永斌,李向文,田珍榛,等. 时间序列分解法在我国食物中毒发病人数预测中的应用 [J].中国卫生统计, 2015,32(4):624-626. 胡建利,刘文东,粱祁,等. 季节指数法和ARIMA模型在感染性腹泻周发病数预测中的应用研究 [J]. 中华疾病控制杂志, 2013,17(8):718-721. 葛哲学. 神经网络理论与MATLABR2007实现 [M]. 北京:电子工业出版社, 2007:111. Wu W, Guo J, An S, et al. Comparison of two hybrid models for forecasting the incidence of hemorrhagic fever with renal syndrome in Jiangsu Province,China [J]. PLoS One, 2015,10(8):e0135492. Zheng YL, Zhang LP, Zhang XL, et al. Forecast model analysis for the morbidity of tuberculosis in Xinjiang, China [J]. PLoS One, 2015,10(3):e0116832. Zhang G, Huang S, Duan Q, et al. Application of a hybrid model for predicting the incidence of tuberculosis in Hubei, China [J]. PLoS One, 2013,8(11):e80969. -
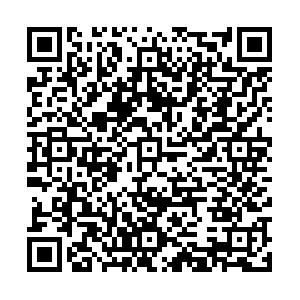
计量
- 文章访问数: 410
- HTML全文浏览量: 72
- PDF下载量: 41
- 被引次数: 0