Predictors of breast cancer screening utilization among female at high risk of developing breast cancer: application of a Lasso Logistic model
-
摘要: 目的 探讨Lasso Logistic回归模型在乳腺癌高风险人群筛查利用相关因素研究中的应用。方法 基于健康风险评估模型筛选乳腺癌高风险人群,利用Lasso Logistic回归模型进行变量选择,通过交叉验证选择模型中的最优调和参数λ,再建立传统Logistic回归模型分析筛查利用情况的影响因素。结果 经健康风险评估模型筛选后,共纳入771名乳腺癌高风险人群,乳腺癌筛查利用率为72.1%。交叉验证选择的最优λ为0.044,经Lasso Logistic回归模型进行变量筛选后纳入的自变量为年龄、文化程度、既往乳腺疾病史和乳房自检行为,赤池信息准则(akaike information criterion,AIC)和贝叶斯信息准则(bayesian information criterion,BIC)分别为762.44和785.68,均低于传统Logistic回归模型(762.73,804.55)。结论 Lasso Logistic回归模型可用于乳腺癌高风险人群筛查利用情况相关因素研究。年龄、文化程度、既往乳腺疾病史和乳房自检行为影响乳腺癌高风险人群的筛查利用情况。
-
关键词:
- Lasso Logistic回归模型 /
- 乳腺癌筛查 /
- 相关因素
Abstract: Objective To evaluate the application of a Lasso Logistic model for potential factors related with breast cancer screening among female at high risk of developing breast cancer. Methods A health risk appraisal model was used to measure women's objective risk of developing breast cancer. Lasso Logistic model was used to analyze the predictors of breast cancer screening utilization. Cross validation method was used to choose λ for Lasso Logistic model. In addition, the akaike information criterion (AIC) and bayesian information criterion (BIC) were chosen to evaluate the model fitting of Lasso Logistic model, compared with full Logistic model. Results Of the 771 women at high risk of developing breast cancer, 72.1% attended screening. The cross validation resulted in λ=0.044 for Lasso Logistic model. Age, education level, personal history of breast disease and breast self-examination were associated with breast cancer screening utilization in Lasso Logistic model and full Logistic model. AIC of Lasso Logistic model and full Logistic model were 762.44 and 762.73, respectively. And BIC were 785.68 and 804.55, respectively. Conclusions Lasso Logistic model was a sound fitting model for predictors of breast cancer screening utilization among women at high risk of developing breast cancer. Age, education level, personal history of breast disease and breast self-examination were predictors of breast cancer screening utilization.-
Key words:
- Lasso Logistic model /
- Breast cancer screening /
- Relative fators
-
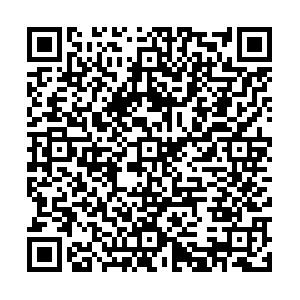
计量
- 文章访问数: 1051
- HTML全文浏览量: 147
- PDF下载量: 120
- 被引次数: 0