Forecasting influenza like illness in Urumqi based on ARIMAX model
-
摘要: 目的 探讨ARIMAX模型(autoregressive integrated moving average model-X,ARIMAX)在流感发病趋势预测方面的效果,为提高此模型在传染病发病预测方面的使用提供依据。方法 收集乌鲁木齐市(乌市)2013年1月~2016年12月的流感样病例(ILI)病例数和大气颗粒物PM2.5及PM10浓度数据,用R软件建立ARIMA及ARIMAX模型,并对2017年前10周ILI病例数做预测。结果 乌市2013年1月~2016年12月ILI病例总数161 773例,周平均发病数为777例;时序图显示呈冬春季高发的特点。流感周发病数建立ARIMA(1,0,0)模型,赤池信息准则(akaike information criterion,AIC)=2 549.03;以大气颗粒物PM2.5及PM10为影响变量,带入转换函数建立ARIMAX模型,AIC=2 535.51,且模型各参数有统计学意义。使用迭代法对前10期(10周)数据进行预测,预测结果显示仅预测3期(3周)误差最小;两模型预测误差百分比绝对值均值(mean absolute percentage error,MAPE)分别为12.019 74%,12.014 17%,显示两模型均有较好的预测精度。结论 ARIMA模型和ARIMAX模型均能较好预测短时间内ILI病例数的发病趋势,为流感监测和预防控制提供依据。Abstract: Objective To explore the effect of auto-regressive integrated moving average model-X(ARIMAX) model on predicting influenza trend in Urumqi, and to provide basis for further application of modeling in forecasting infectious diseases. Methods Data on the number of influenza-like illness(ILI) cases and atmospheric particulate matter(PM2.5 and PM10) concentrations were collected from January 2013 to December 2016 in Urumqi. We used R to construct auto-regressive integrated moving average (ARIMA) model and predicted the number of ILI cases in the first 10 weeks in 2017 using ARIMAX model. Results From January 2013 to December 2016, the total number of ILI cases was 161 773 and the number of average weekly ILI cases was 777. The time series of ILI cases indicated that there was a high prevalence during winter and spring. ARIMA (1, 0, 0) model was established based on weekly ILI cases and akaike information criterion (AIC)=2 549.03. PM2.5 and PM10 were considered as parameters to build ARIMAX model (AIC=2 535.51) and they were shown to be statistically significant. Using iterative method to predict the data of the first ten periods (i.e. 10 weeks), the results indicated only 3 period (i.e. 3 weeks) prediction had the minimal error.The mean absolute percentage error (MAPE) of the two models was 12.019 74% and 12.014 17% respectively, suggesting both of them had a good predictive accuracy. Conclusions ARIMA model and ARIMAX model can predict trend of ILI cases incidence in short time and provide evidence for influenza monitoring and prevention.
-
Key words:
- Influenza,human /
- ARIMAX model /
- Co-integration /
- Time series
期刊类型引用(11)
1. 赵盼盼,张迷,焦力宾. 基于ARIMAX模型的中国税收收入预测与分析. 高师理科学刊. 2024(05): 22-27 . 百度学术
2. 王向前,吴东隆,郑健彤. 货物吞吐量预测的改进ARIMAX方法——以天津港为例. 运筹与管理. 2022(03): 138-144 . 百度学术
3. 王升,杨金兰,陈瑞,陈建华,刘如品,杜秋争,荆自伟. 求和自回归移动平均模型与动态回归模型预测产超广谱β-内酰胺酶肺炎克雷伯菌的检出率. 西北药学杂志. 2022(02): 159-165 . 百度学术
4. 翟梦梦,李国华,高雪芬,王旭春,任浩,李美晨,全帝臣,罗天娥,赵晋芳,陈利民,仇丽霞. 基于奇异谱分析的ARIMA模型在山西省流感预测中的应用. 现代预防医学. 2021(09): 1550-1555 . 百度学术
5. 姚秀侠,杜洁. ARIMAX模型在A省人口出生率预测中的应用. 衡阳师范学院学报. 2021(03): 33-39 . 百度学术
6. 梁达,商越,王兆芬,马斌忠. 青海省肺结核发病与气象因素的时间序列分析. 中华疾病控制杂志. 2021(10): 1186-1193 . 本站查看
7. 龚风云,王凯,樊旭成,杨建东. 乌鲁木齐市流感样病例与气象因素的ARIMAX模型预测分析. 公共卫生与预防医学. 2020(02): 4-8 . 百度学术
8. 吴凤华,胡澄,江泽标,田娟. ARIMA预测模型在电力供需预测中的应用. 能源工程. 2020(04): 82-87+98 . 百度学术
9. 赵金华,石燕,曹海兰,李永红,李红,卢囡囡,徐莉立. 高原地区青海省2017-2018年度流感样病例流行病学特征分析. 中华疾病控制杂志. 2019(01): 39-44 . 本站查看
10. 傅伟杰,谢昀,曾志笠,刘晓青. 三种模型在江西省流感样病例预测中的应用与比较. 中华疾病控制杂志. 2019(01): 101-105 . 本站查看
11. 龚风云,樊旭成,杨建东,王凯. 乌鲁木齐市气象因素对流感样病例的影响. 职业与健康. 2019(16): 2263-2266 . 百度学术
其他类型引用(12)
-
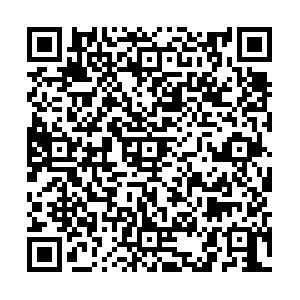
计量
- 文章访问数: 881
- HTML全文浏览量: 155
- PDF下载量: 53
- 被引次数: 23